memory metadata
Livia Foldes
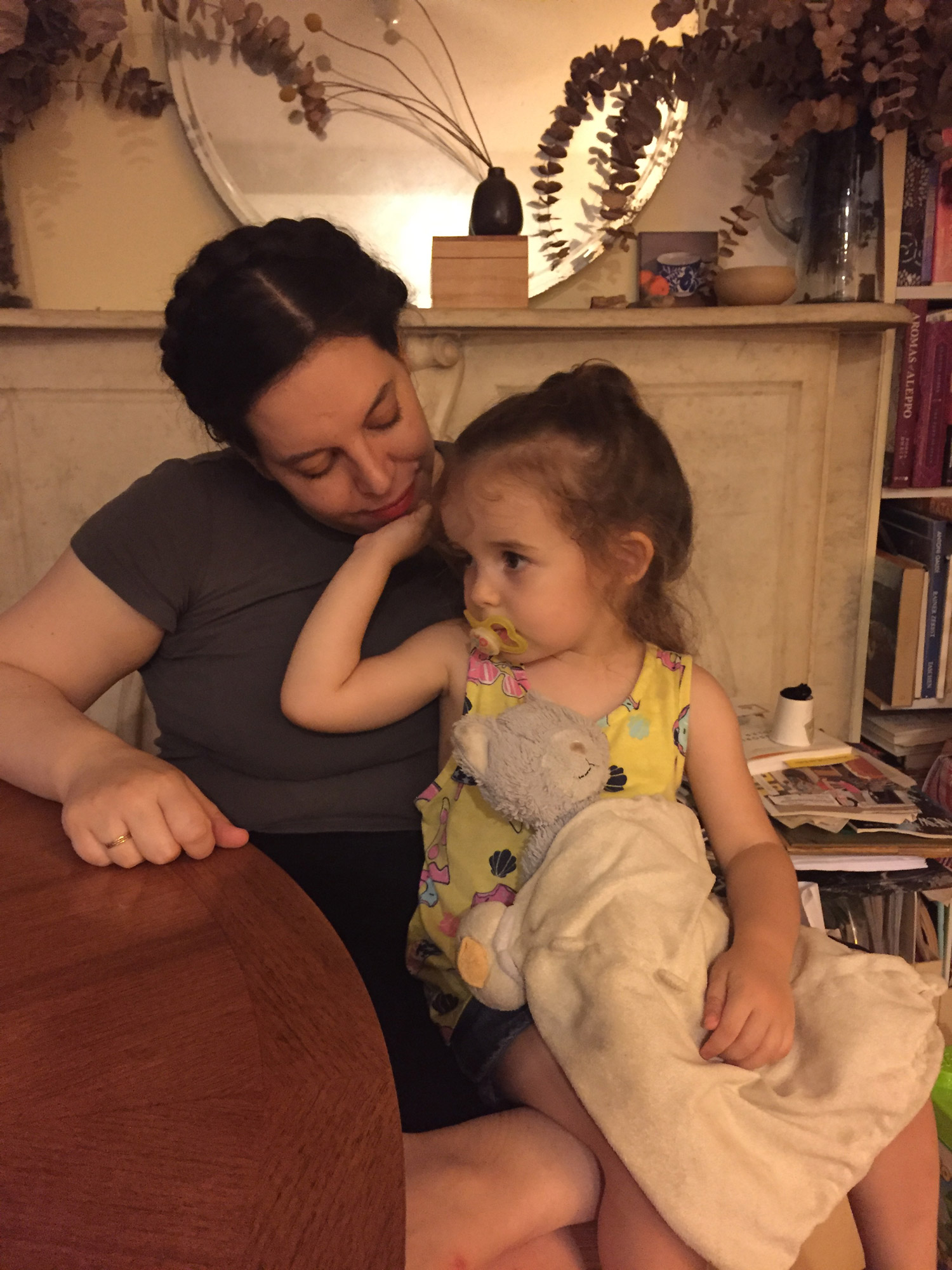
This is a photograph I took of my sister holding my niece.
I took it at her apartment, just after I moved to New York last summer. When I look at it, I remember the thick feeling of the humid August air. I see my sister’s tender expression, and how much my niece has grown in the months since then.
1
pixels
But there are many other ways to see this photo.
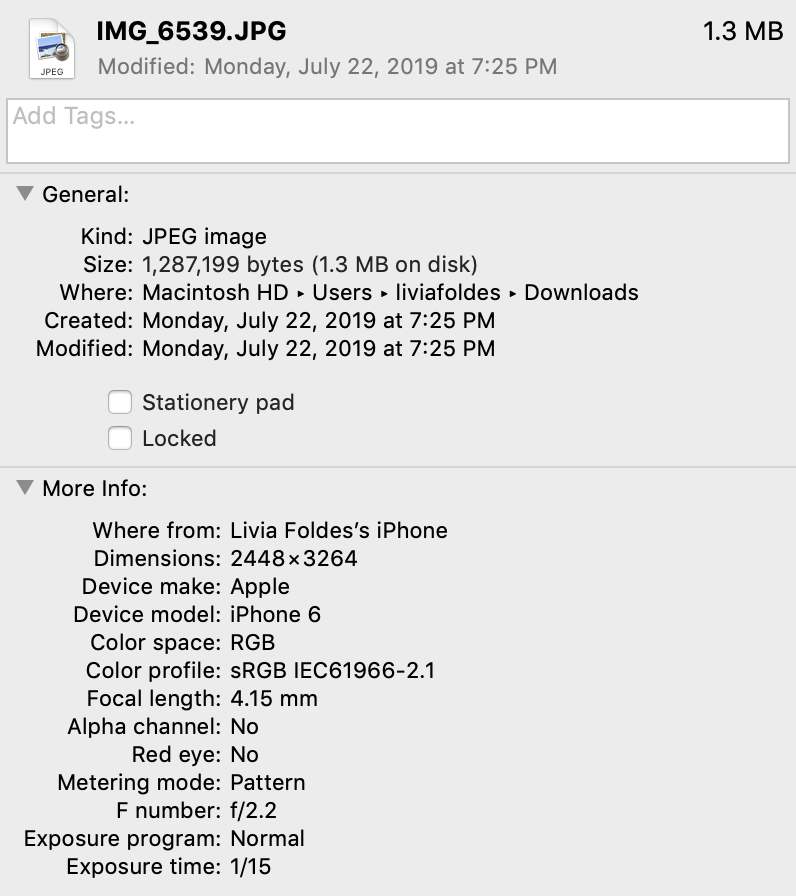
When I ask my Macbook’s operating system to ‘Get Info,’ I can see when it was taken, the device it was taken on, and more. I can see that its 7,990,272 pixels use 1,287,199 bytes of memory.
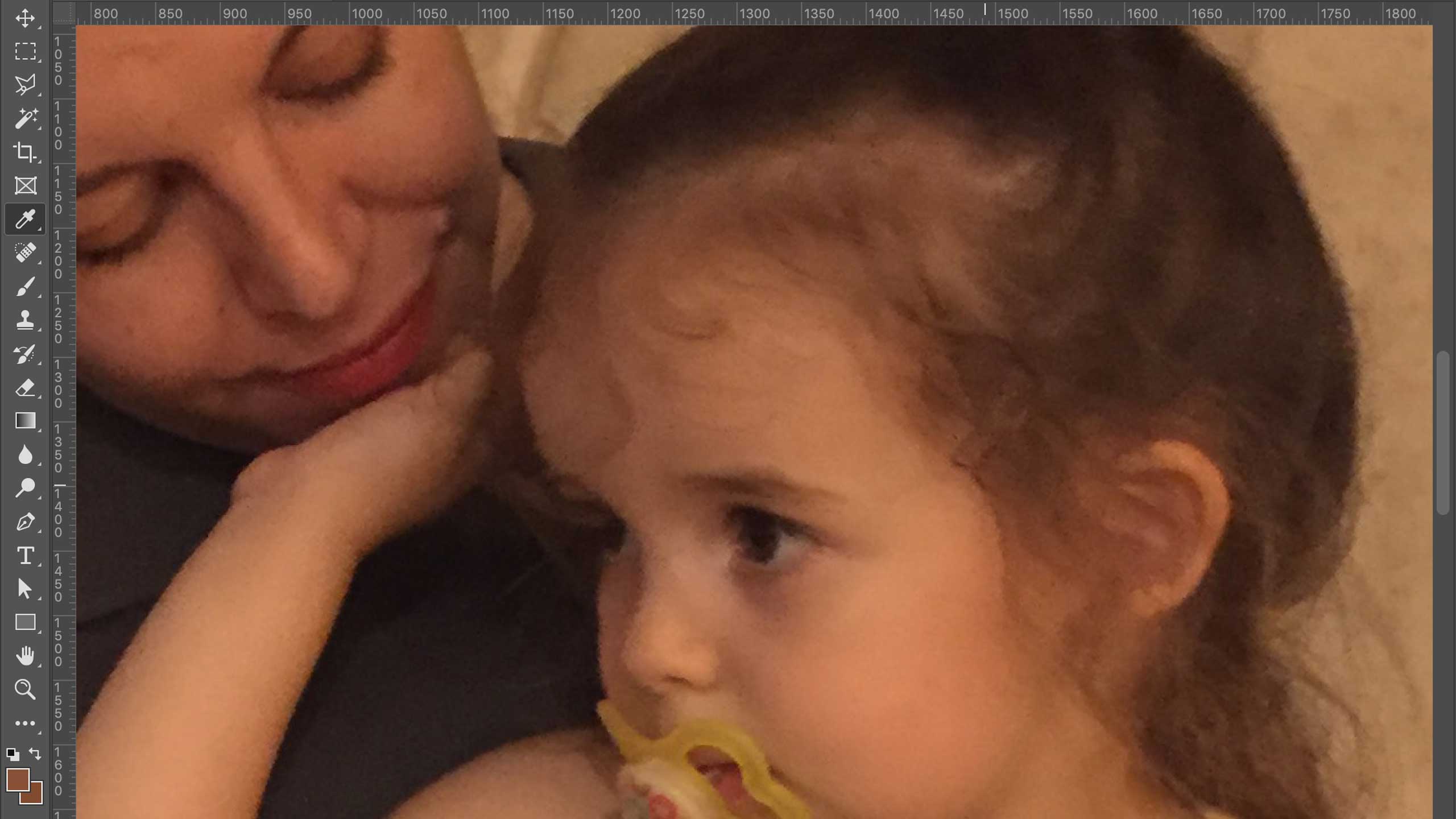
I can open the photo in Photoshop and enlarge it until I see the places where the algorithm in my iPhone’s camera guessed at the colors it couldn’t see in the living room’s dim, late afternoon light.
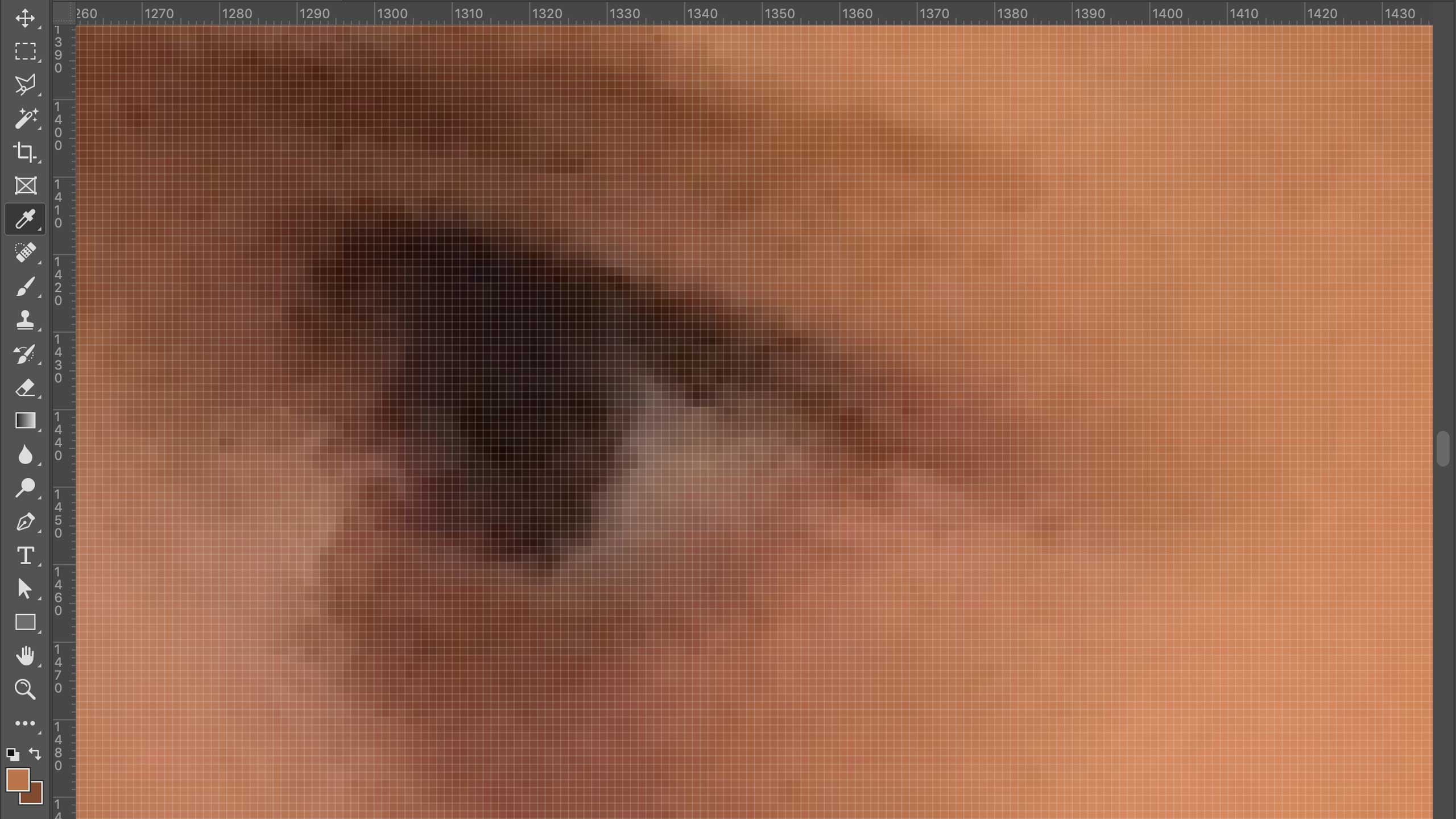
I can zoom in until I see the individual pixels.
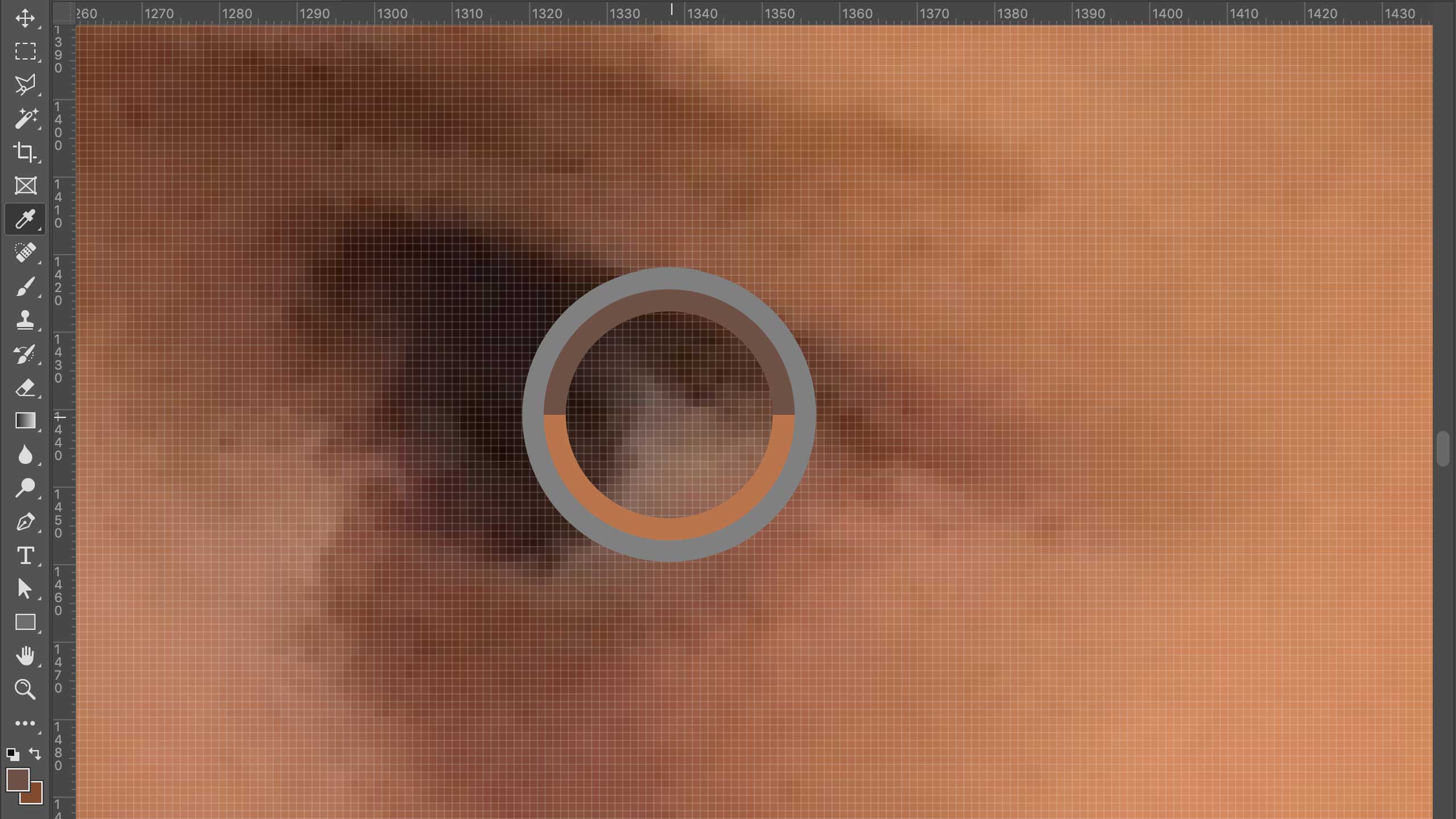
I can select a single pixel,
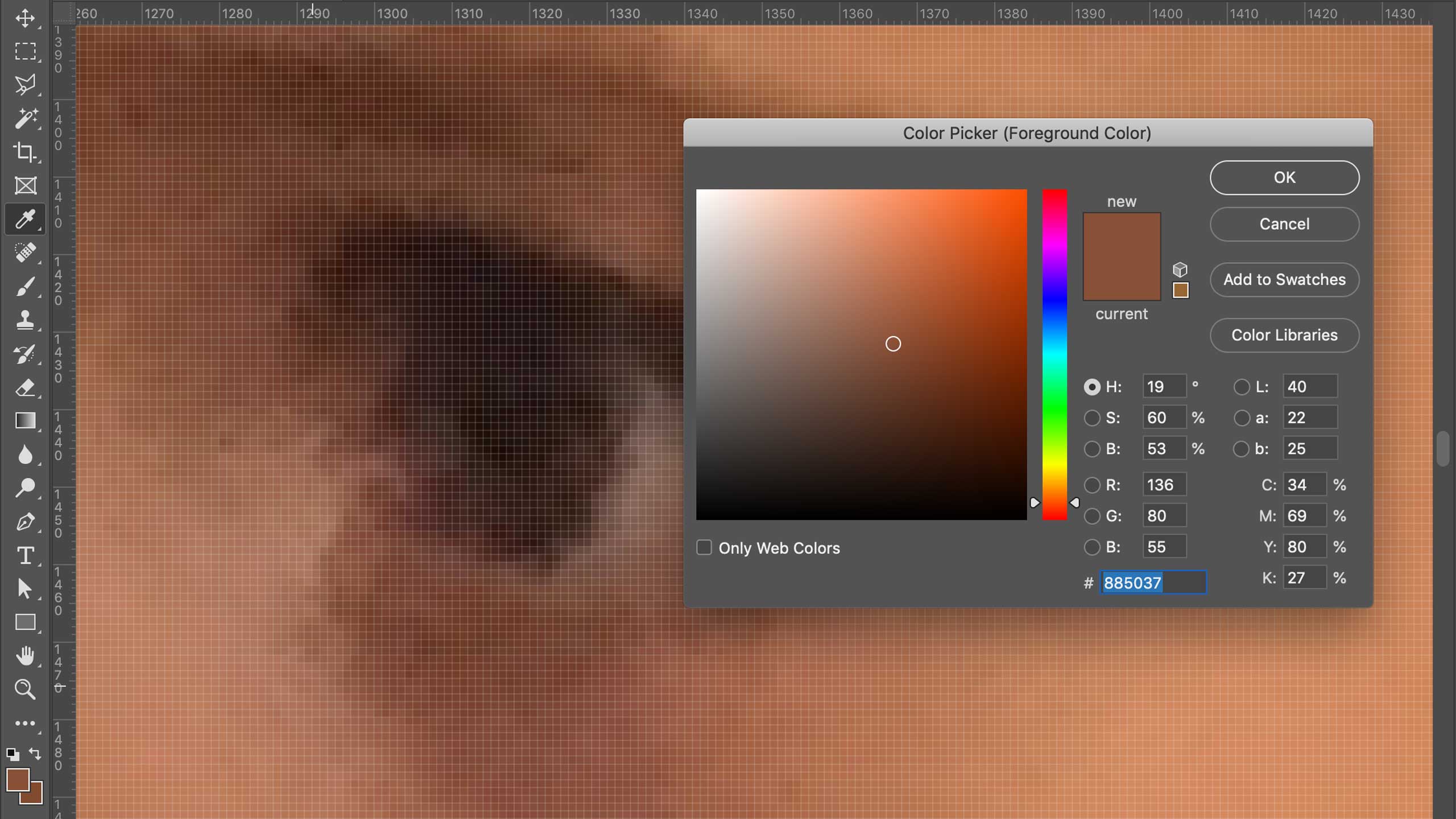
and see the different formulas my computer uses to translate that pixel’s data into color.
2
place
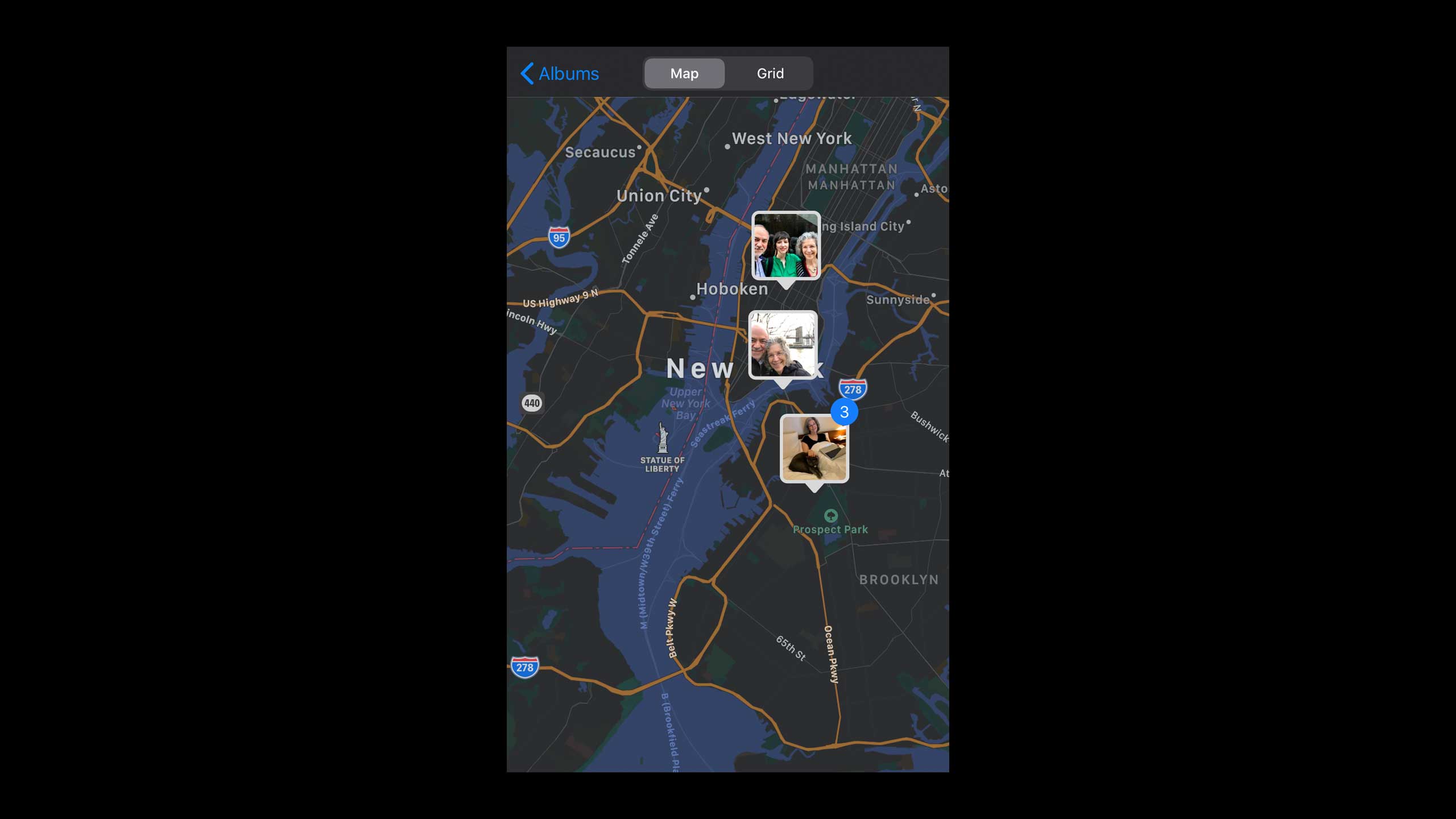
My iPhone remembers where I took the photo.
In Google Maps, I can see a photograph of the exact spot.
The photograph has so many pixels that I can see my sister’s living room where I took the photo.
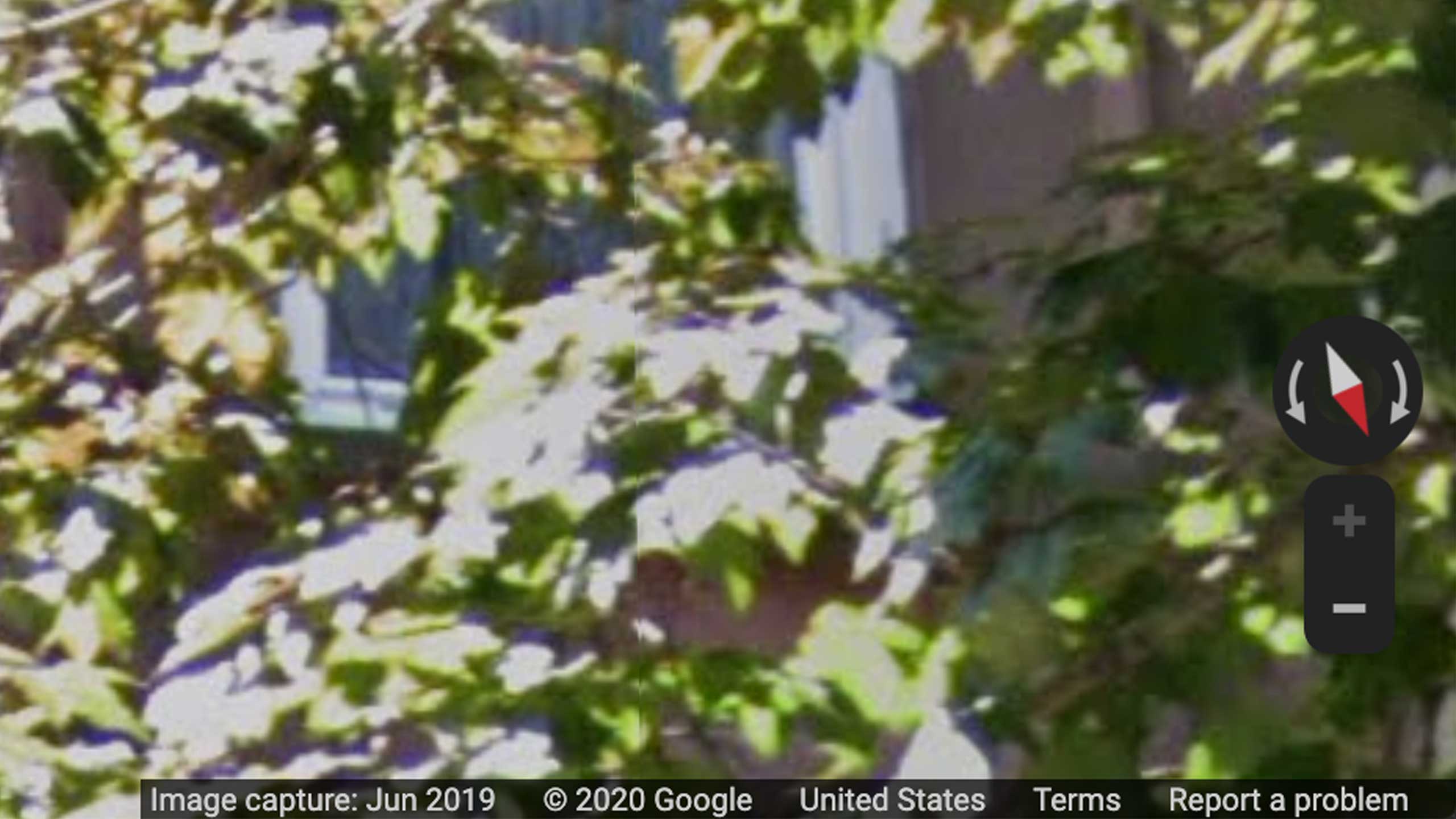
This photo has metadata, too. It was taken by an employee of Google in June, 2019.
Over the last eleven years, someone has photographed her street eight times, at semi-regular intervals.
Google’s maps, the surveillance they facilitate, and the wealth they generate have remade cities like Brooklyn. Its Street View photographs are at once artifacts of the mapping process, documents of gentrification, and a memory bank of the spaces lost in its wake.1
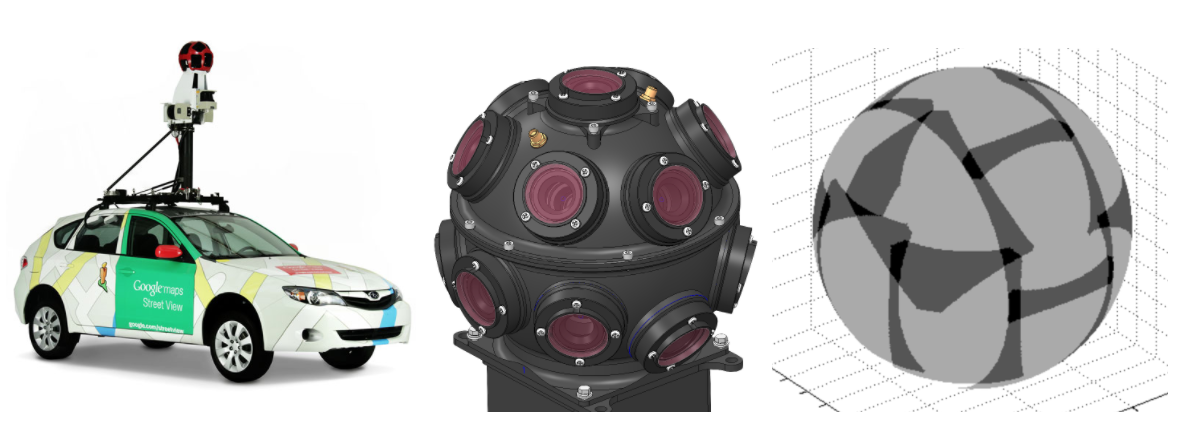
Credit: Google AI blog
The anonymous photographers who take these images were likely hired through an ad on Craigslist, and were likely paid $15-16/hour to drive a car like this one.2
I found this image on Google’s AI blog, in a 2017 post written by people whose job titles are “Software Engineer” and “Research Scientist, Machine Perception.” The blog and its authors point to Google’s motivation for capturing, storing, and publishing a decade of memories of my sister’s street. The photographs are, in fact, “leftovers that happen to be images”3—the visual artifacts of a massive data set used to train artificial intelligence systems to “see” our world.4
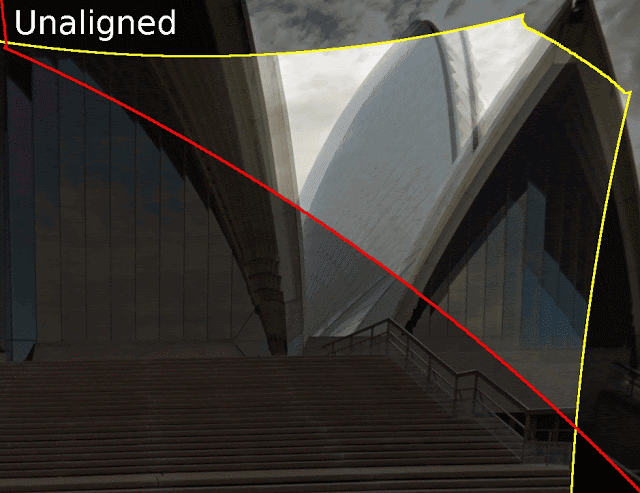
Credit: Google AI blog
The rectangular boundaries of the images I pulled from Street View are arbitrary; the engineer and the research scientist demonstrate the algorithms they use to eliminate “visible seams” from this boundless digital representation of our world.
The post was published the same year Google added 3D depth sensors to the “rosette” of cameras mounted to its Street View cars.5
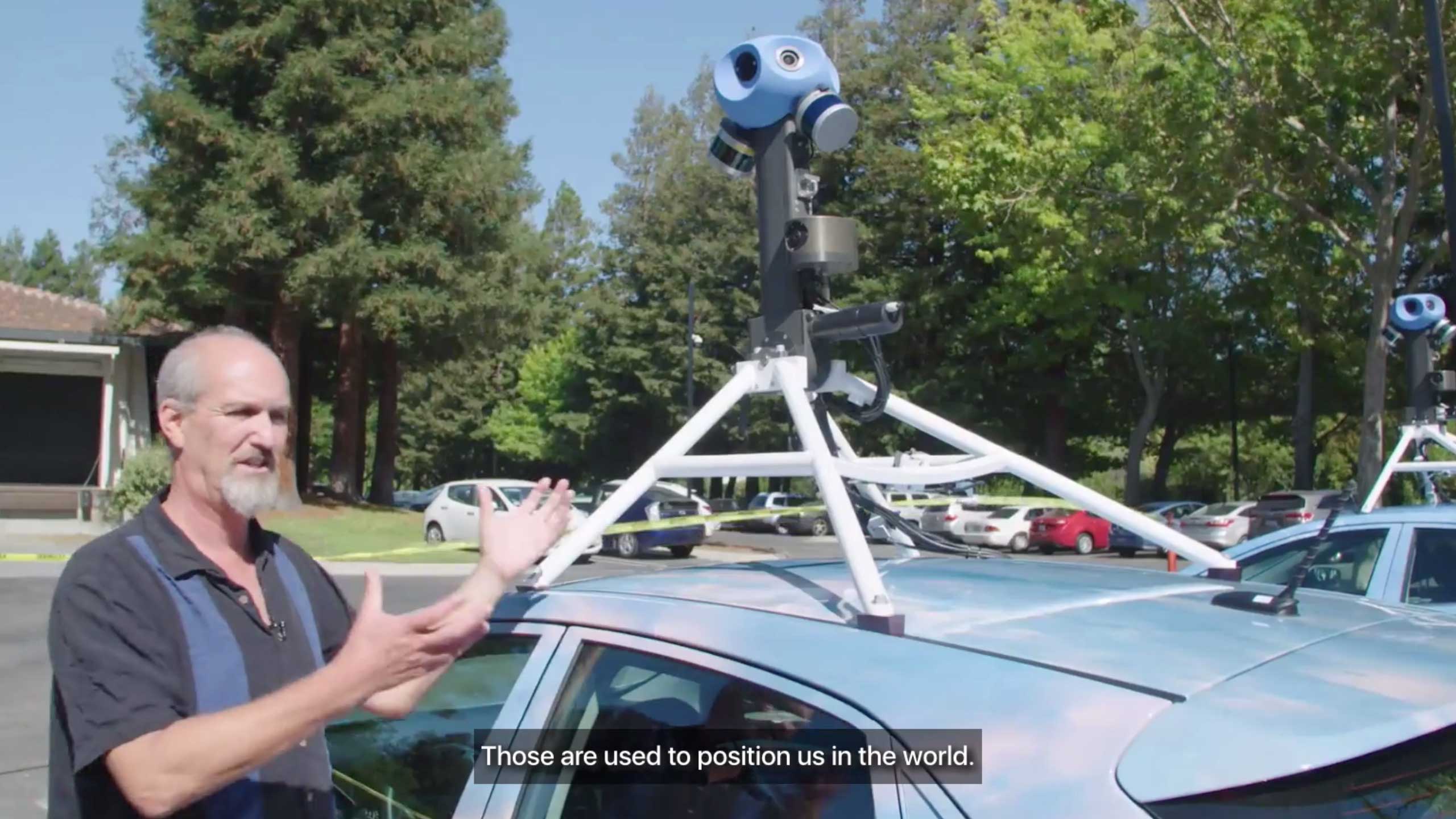
Credit: Wired
These sensors are used, they explained, not just “to position us in the world,” but to “build a deeper data set of knowledge.” They are amassing this knowledge “so that when people come to Google and ask questions about the world around them, we have more and more of the answers.”6
3
vision
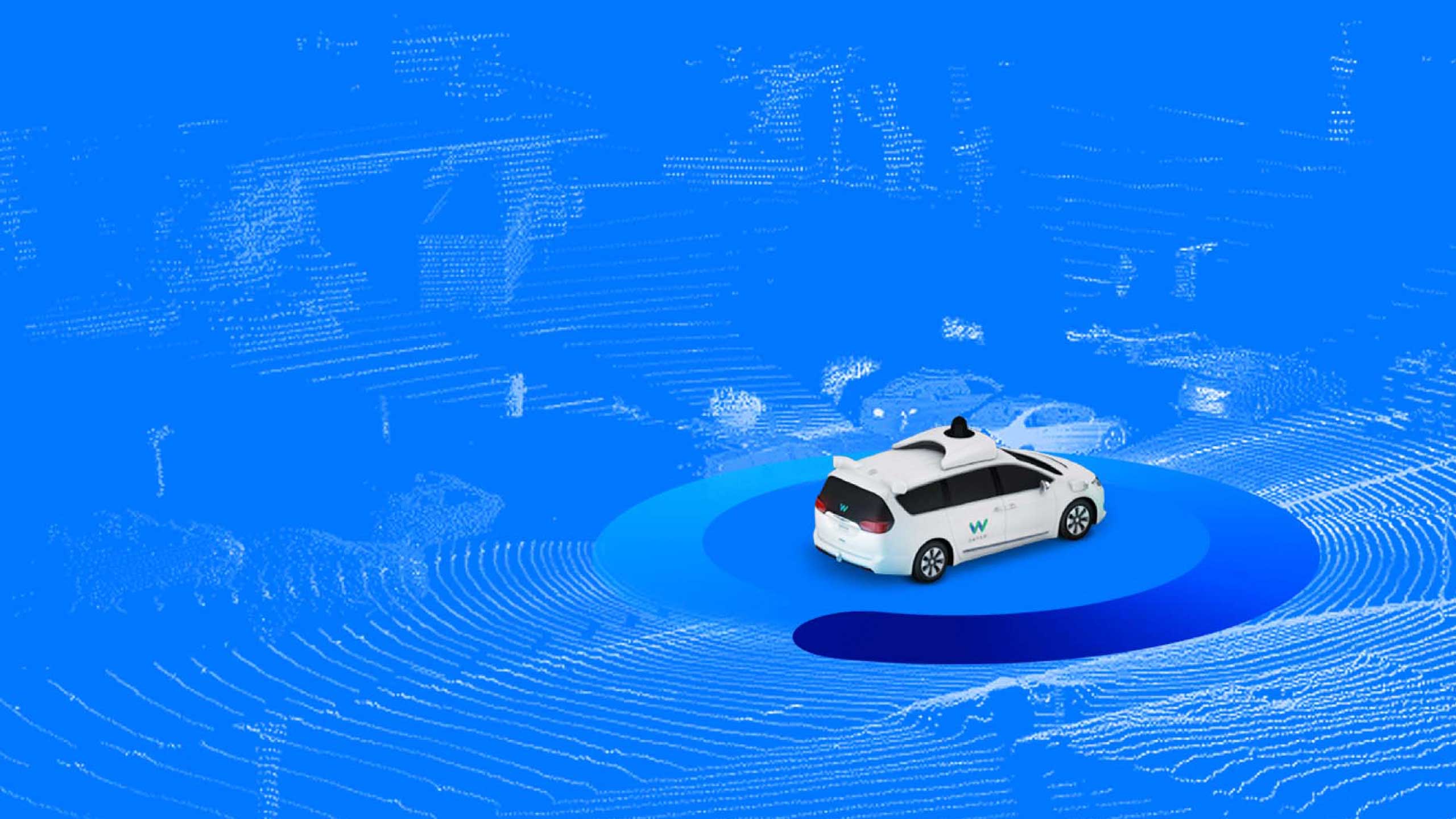
Credit: Waymo
In its website’s FAQs, Waymo (a subsidiary of Alphabet) describes itself as a “self-driving technology company… [built] on technology developed in Google’s labs since 2009”—the year my sister’s apartment was first photographed.
Waymo’s website features a scrolling animation purporting to show us what its cars see. As I scroll, it raises and answers a series of existential questions.
Over an image of a strip mall in Southern California, it asks: Where am I?
What’s around me?
What will happen next?
What should I do?
The visualization imagines a world made simple and legible—a world where my car can answer these questions for me.
As I scroll, the street scene is overlaid with streamlined blue paths, bubbly icons, and text labels. Oncoming cars announce their velocity and distance from me. In this world, labels are definitive and meanings are fixed. This world erases the labor of the humans paid to categorize the millions of photographs that taught the car to see.7
A lone pedestrian, in a box shaded a cautionary orange, stands out from the blue-tinged cars.
The orange gradient is a graphic flourish meant for my eyes, not the car’s sensors. It is there to remind me, perhaps, that Waymo’s algorithmic vision is safe—that it sees humans differently than machines or stop lights.
And it does.
4
recognition
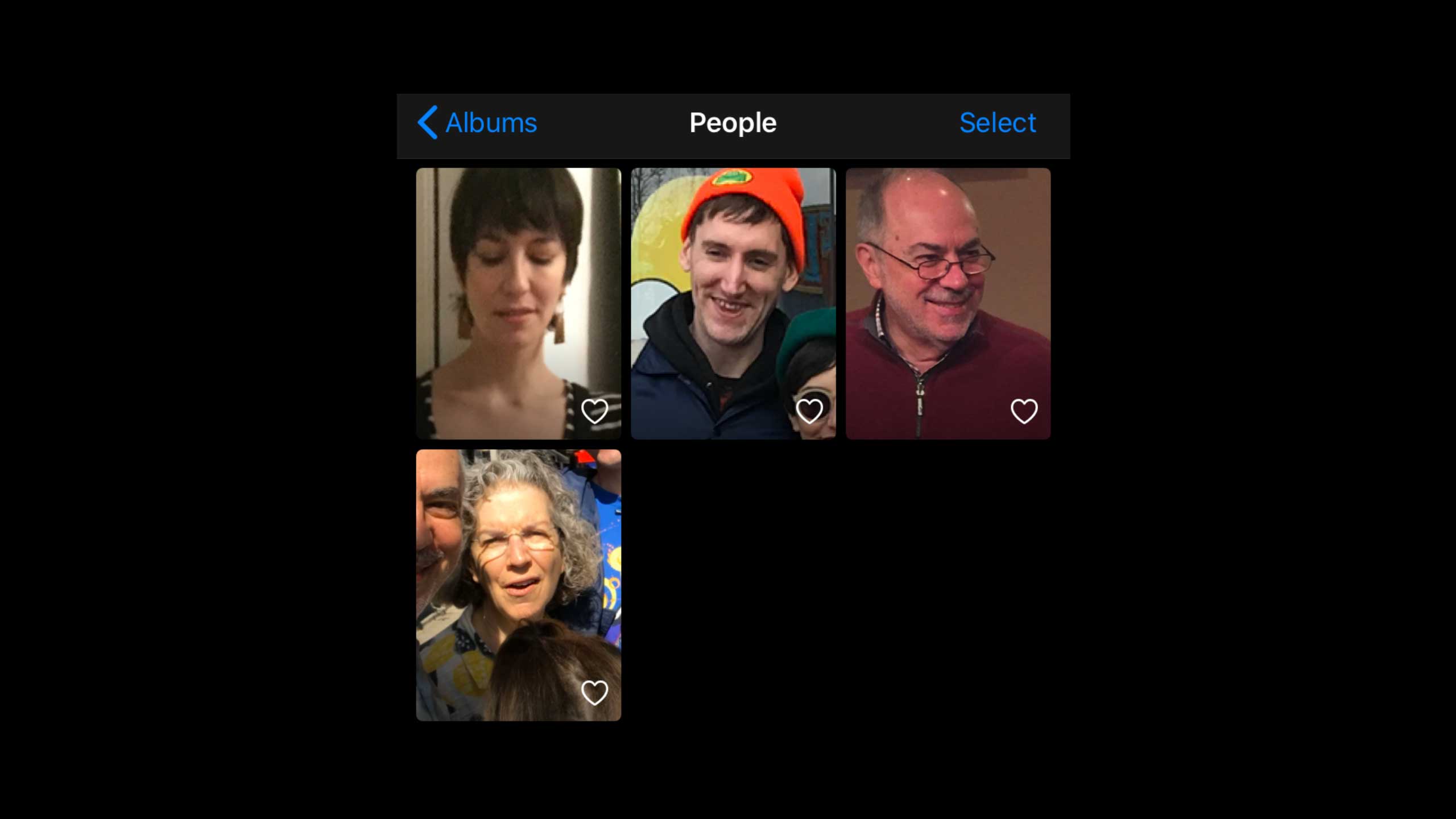
My iPhone’s photo app has trained itself to recognize the people I photograph most often.
According to Apple, it does this by executing 1 trillion operations.
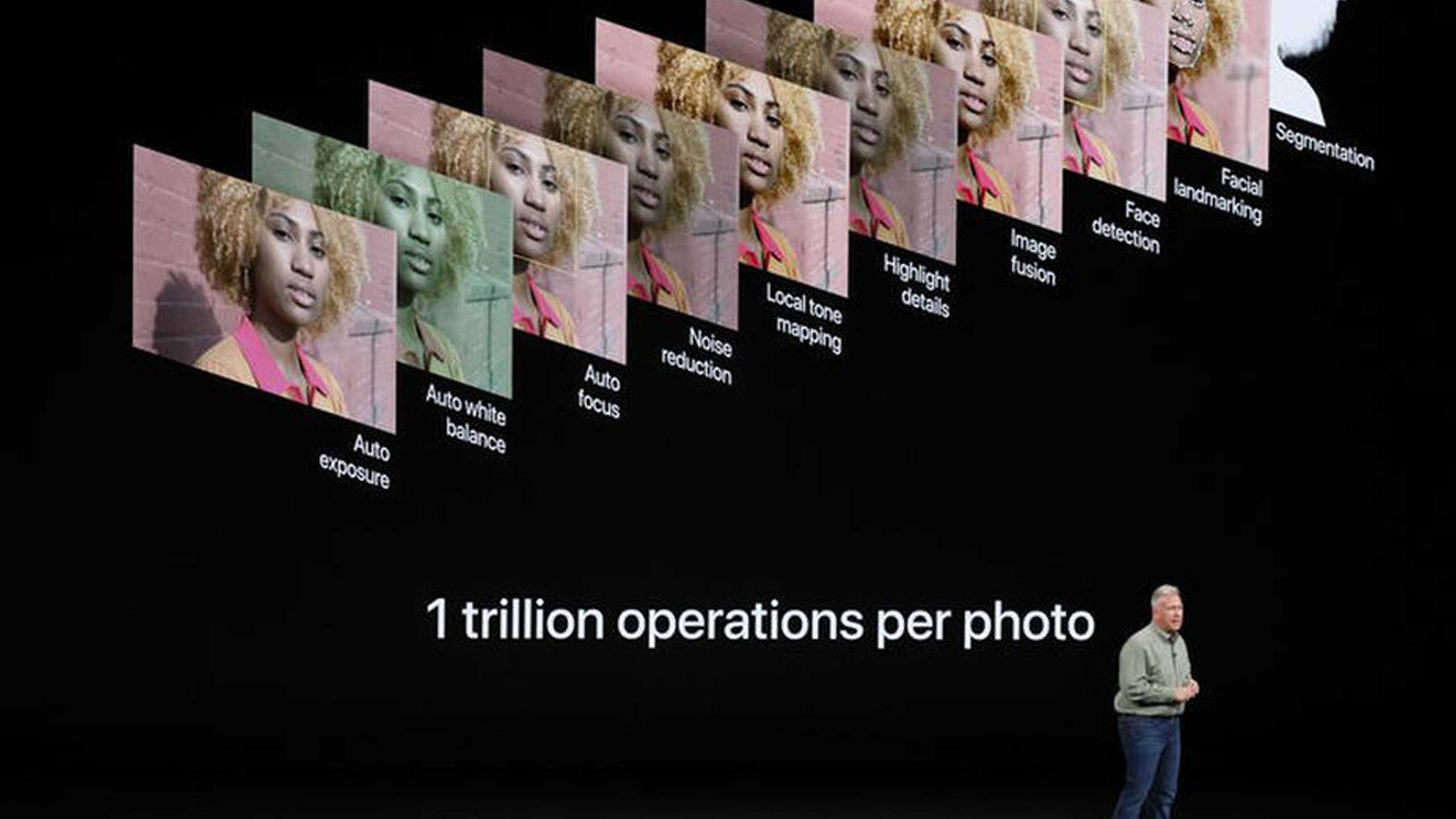
Credit: James Martin/CNET
Many of these operations involve facial detection and analysis. These algorithms are a way for our machines to look at—and, more importantly, categorize—us.
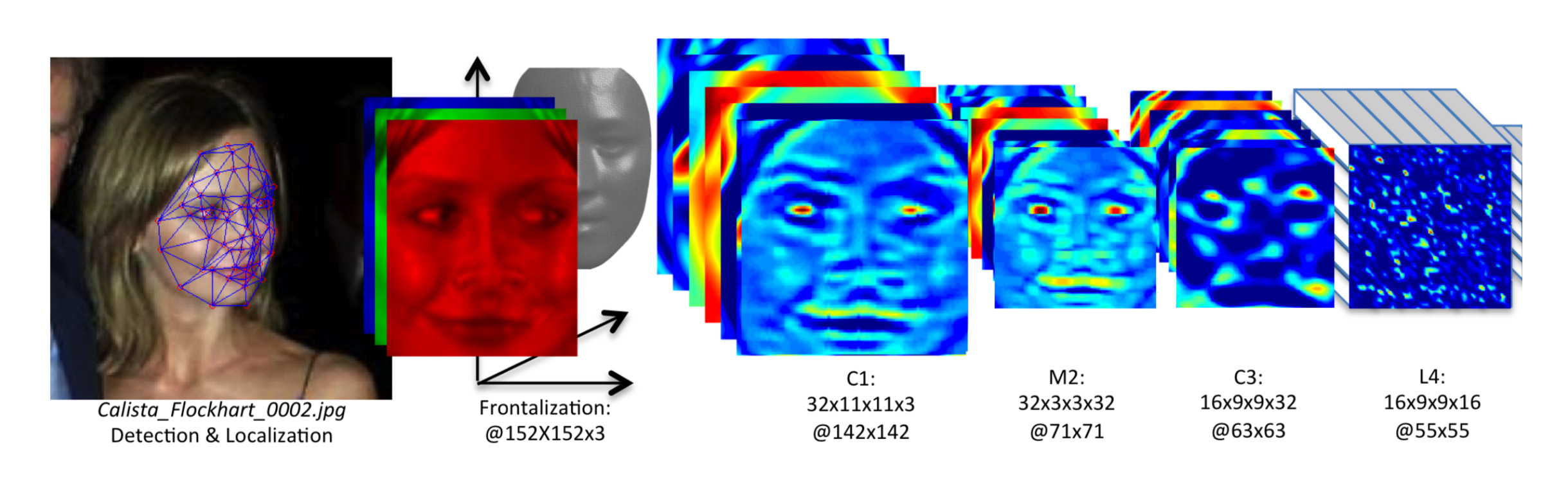
Credit: Facebook Research
Calista Flockhart’s face becomes data, and the data is reconstituted into the label, ‘Calista Flockhart.’
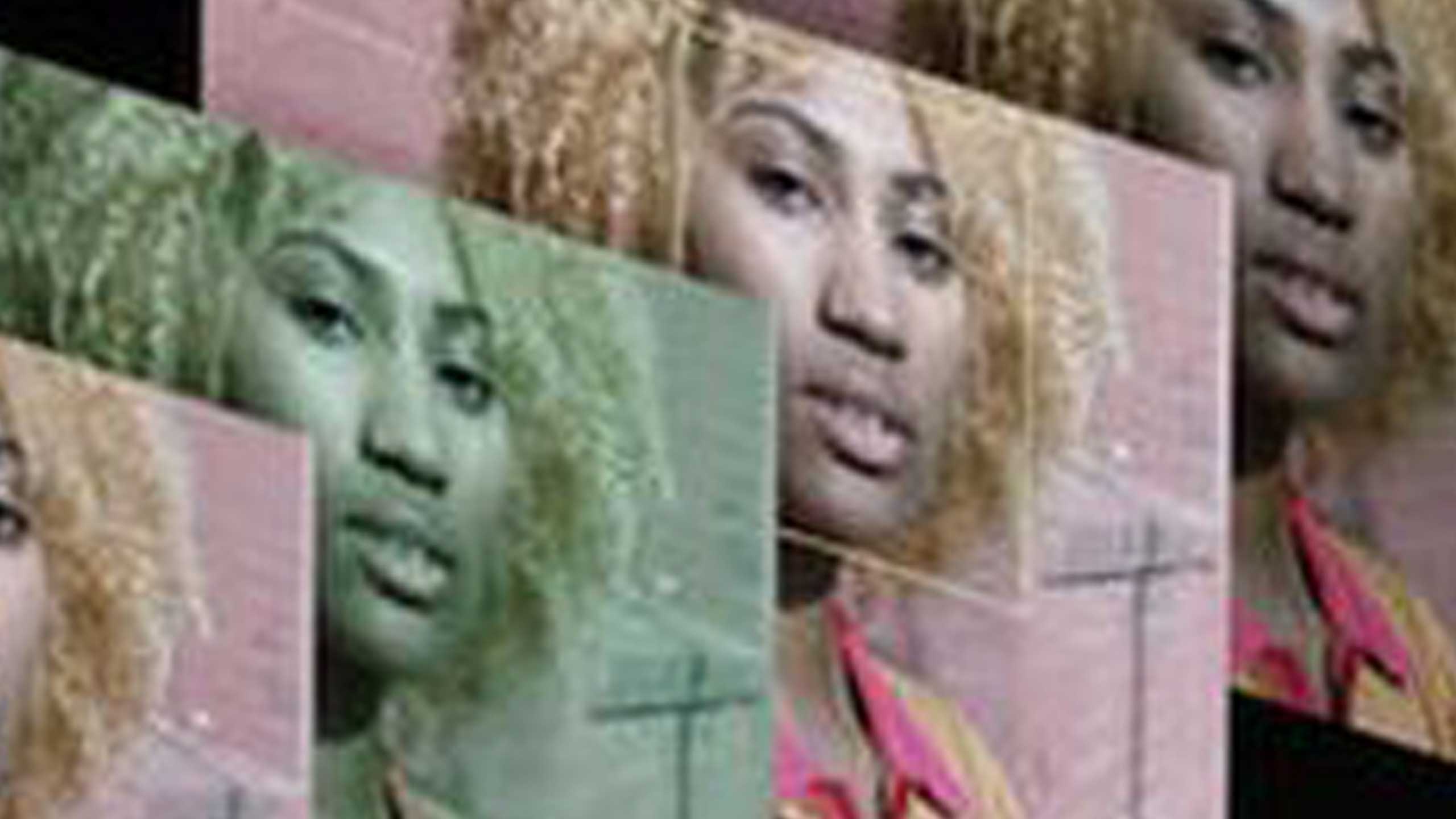
Apple’s decision to illustrate this with an image of a Black woman was not accidental.
It was, perhaps, a nod to AI systems’ well-documented failures to categorize people with dark skin as human.8
5
interpretation
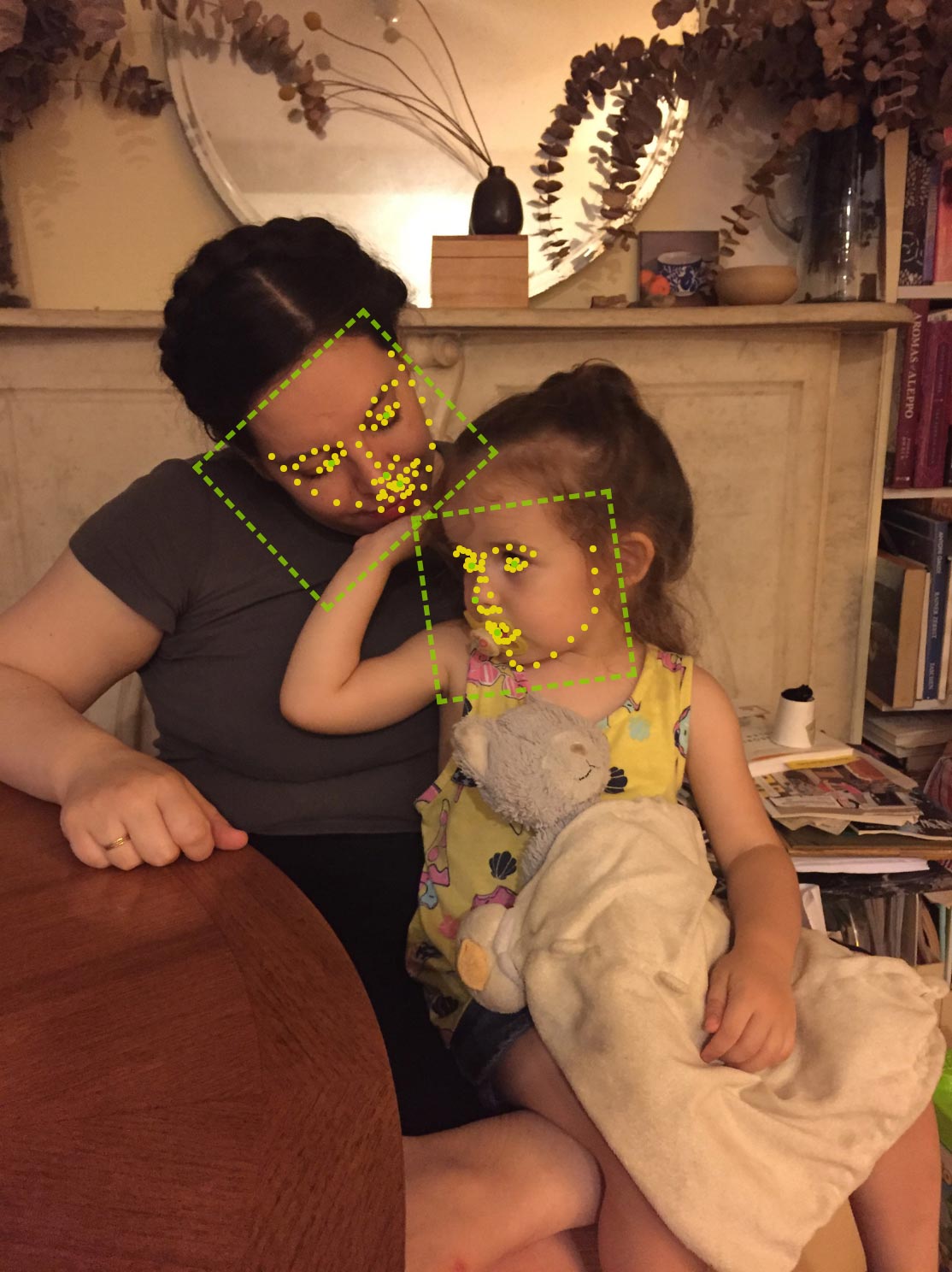
Facial recognition software has no trouble recognizing my sister and niece.
After all, its inventors trained it on images just like this one.9
This photograph that I took to remember a specific time and place looks vastly different to me than it does to a machine. Soon, the machine will be able to see much more of what I see. But our readings will never be the same.
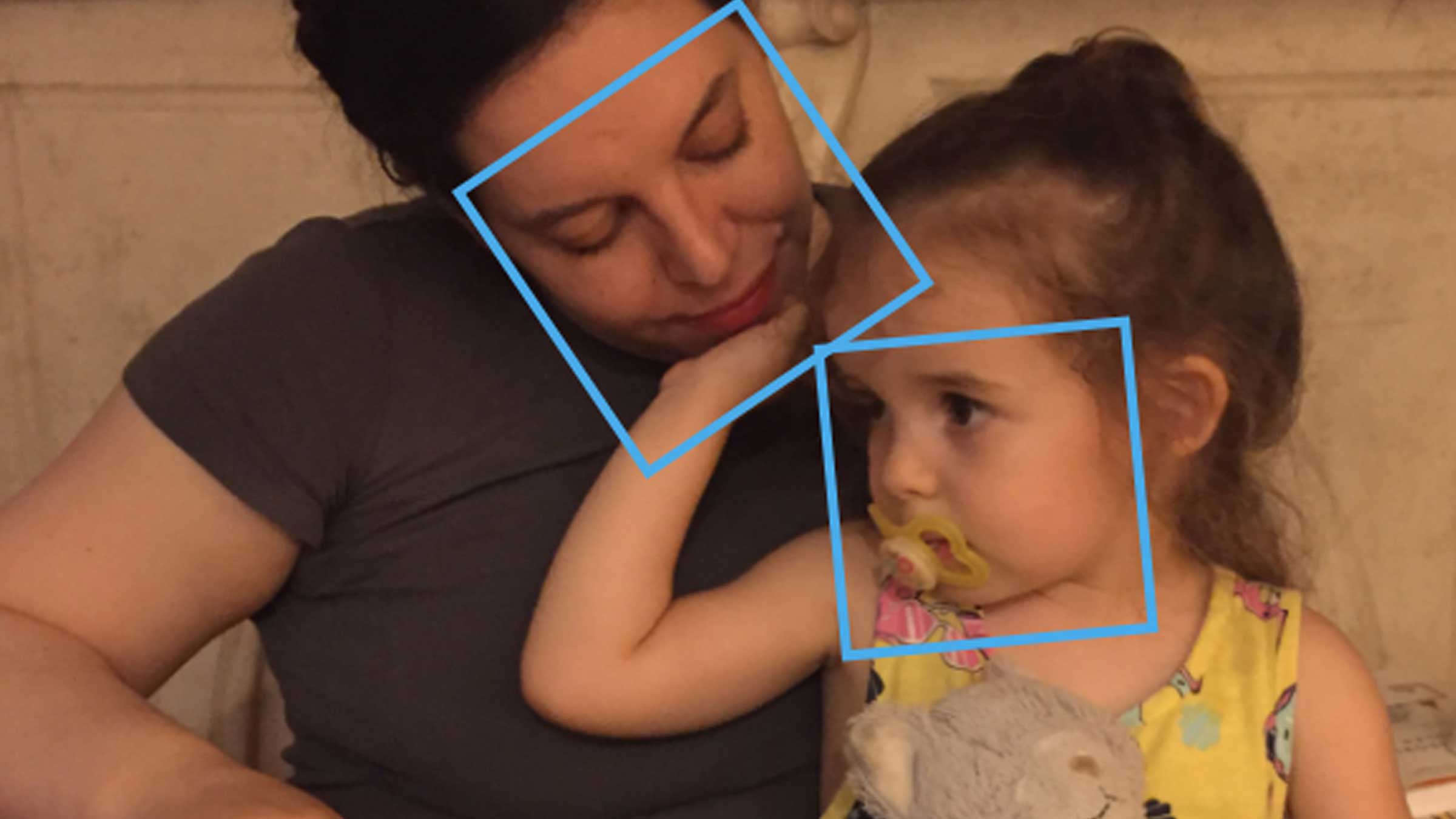
Above: Face detection demo result from Face ++
The machine and I interpret the photograph’s use and meaning in accordance with our distinct cultures, histories, and logics—mine informed by my own experiences, the machine’s determined by its engineers and the companies they work for.
My memory, in the moment of its capture, was at once flattened and augmented, made both less and more.
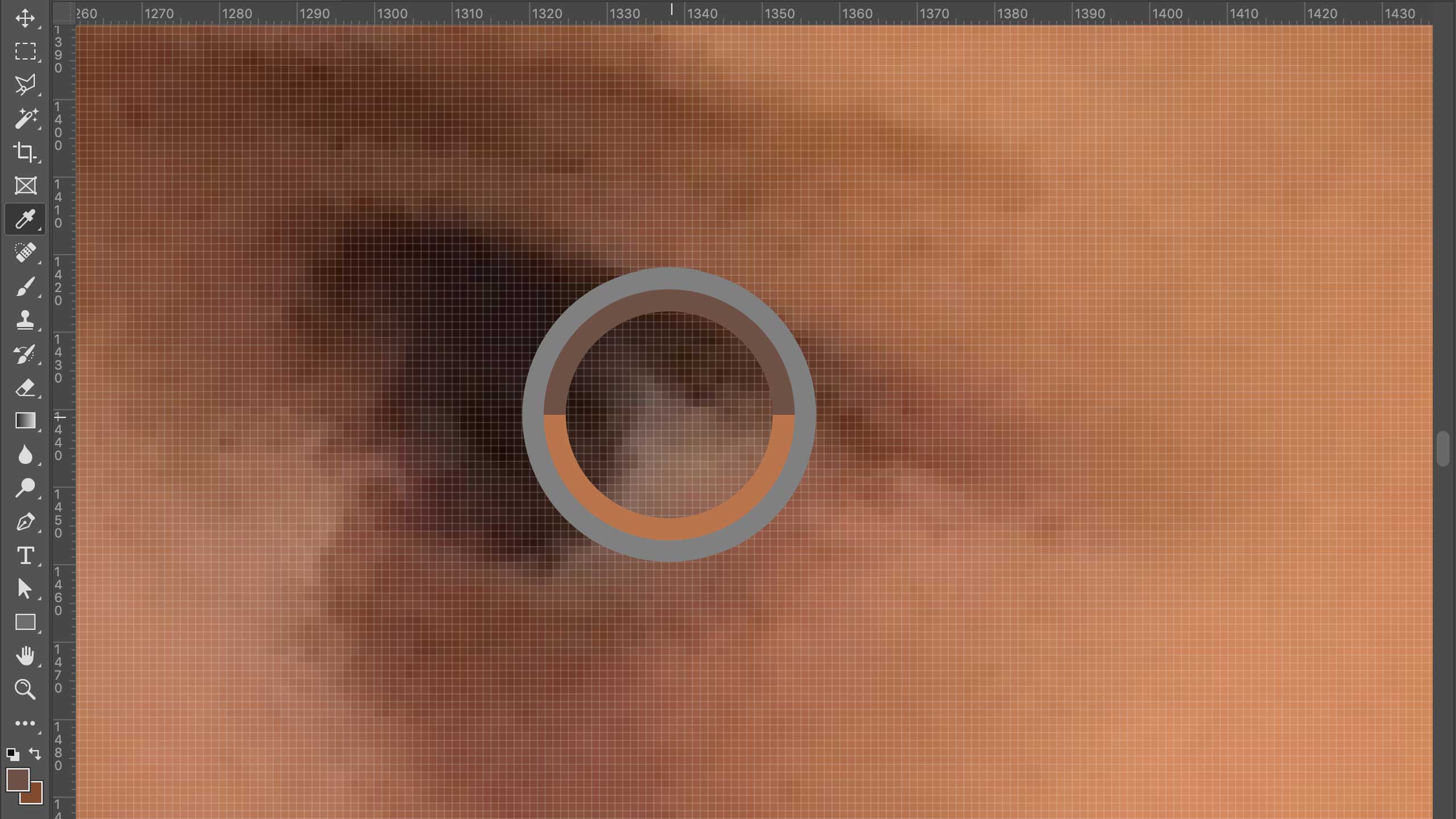
The pixels holding my memory are as unique as an unrepeatable moment, and as infinitely replicable as binary code.
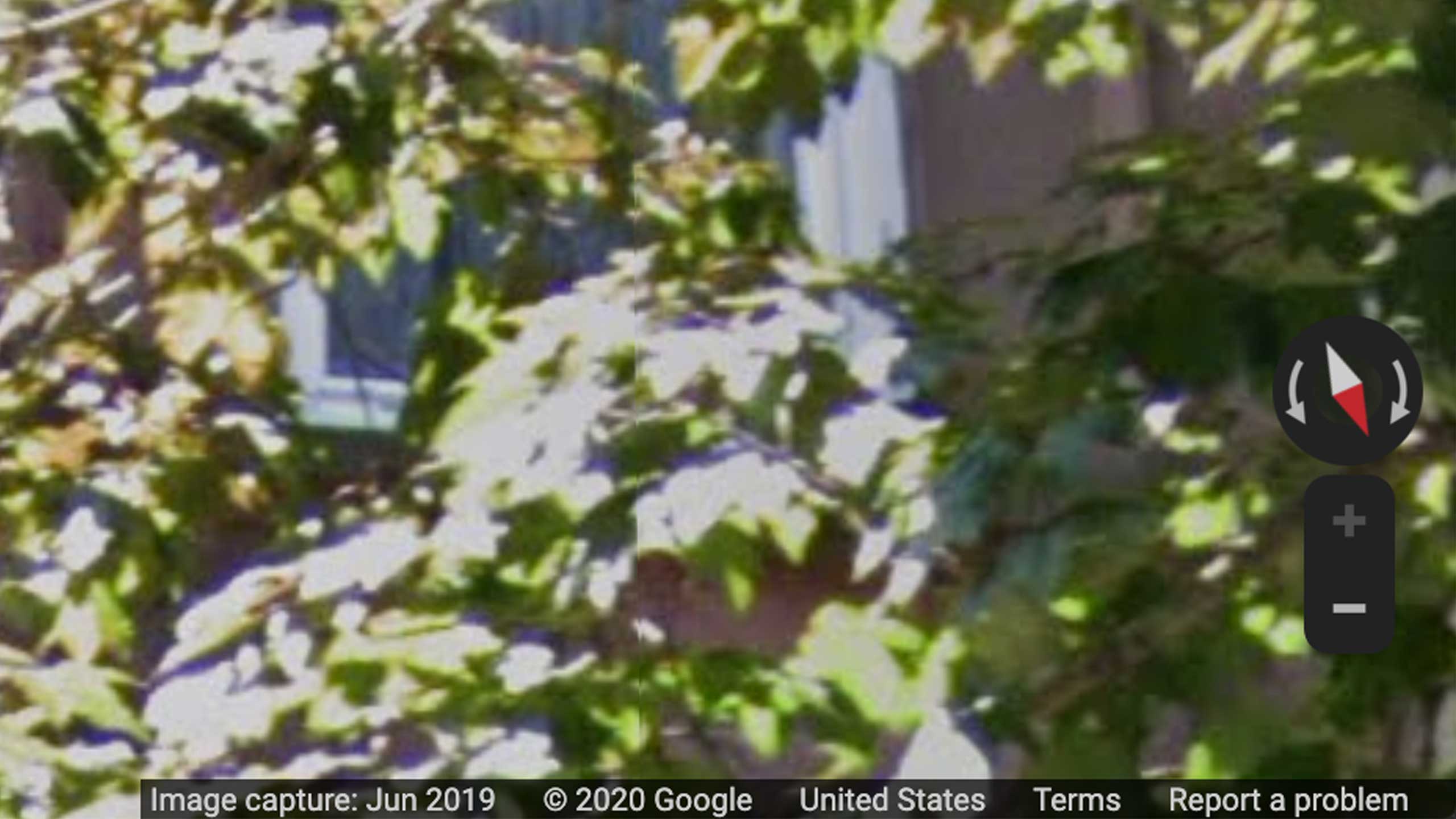
They are situated in space, and distributed across global networks.
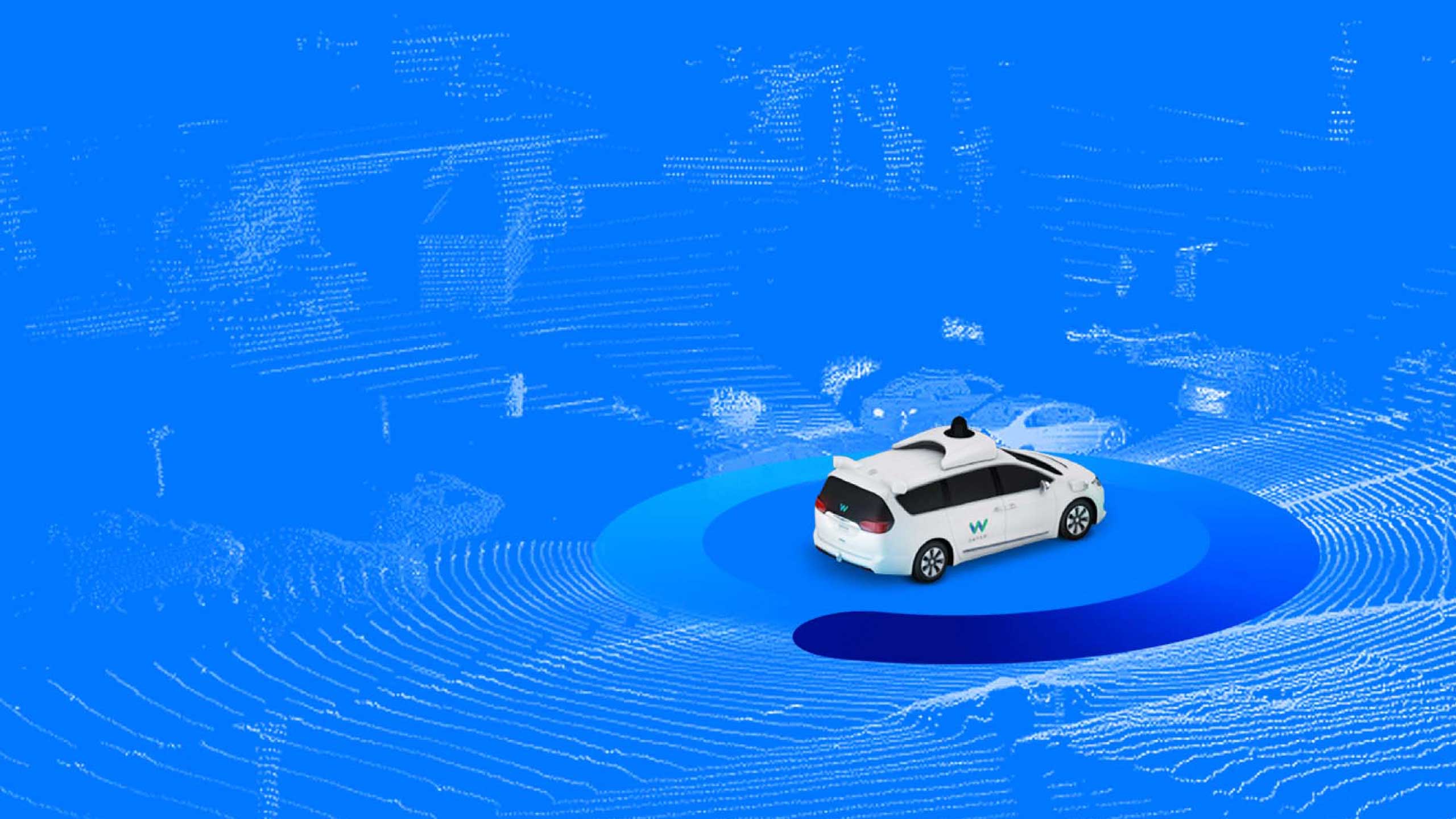
My memory’s metadata doesn’t (yet) contain the texture of my sister’s table, but it can locate her table in relation to the rest of the world.
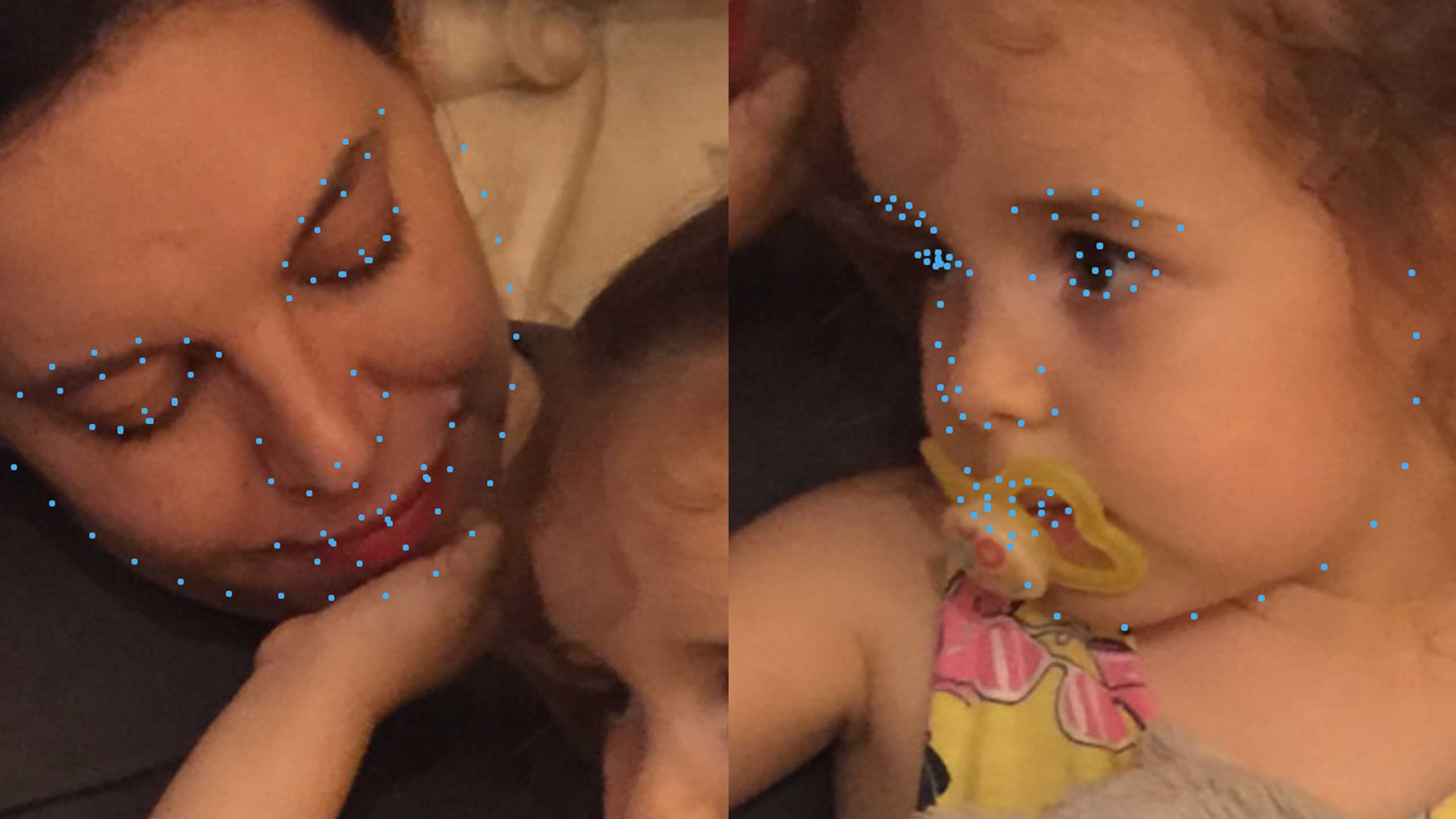
In this layered artifact, my niece and sister are rendered unknowable, even as they are intimately known.